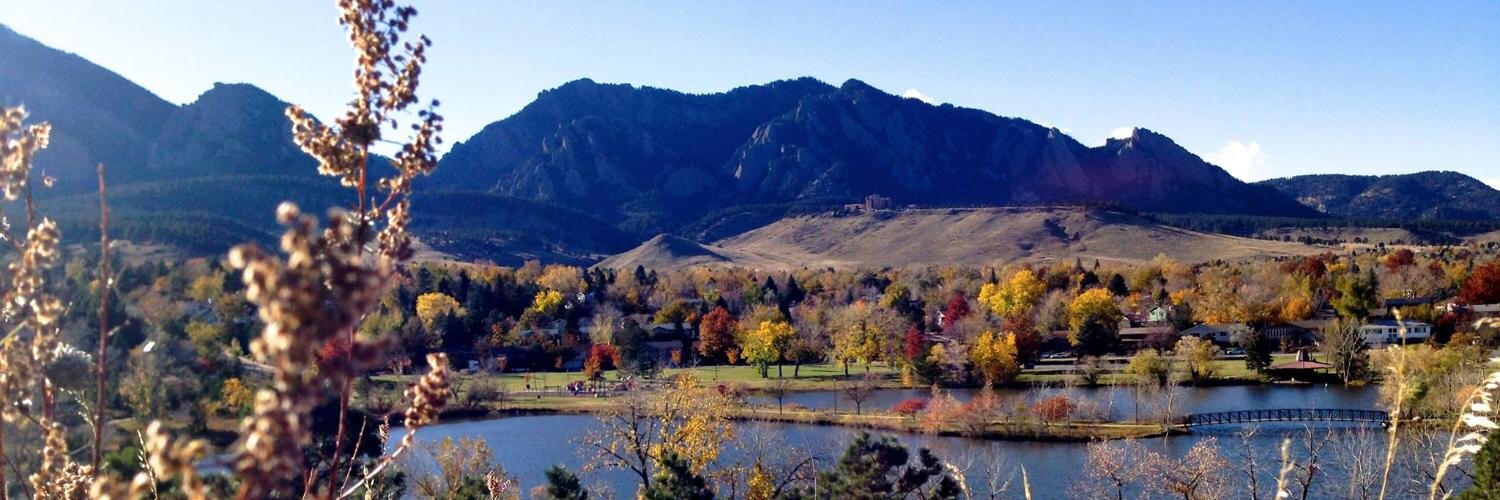
My interests are in Robotics, Artificial Intelligence, and Tangible Interaction Design mostly surrounding systems with multiple agents. I've done other work in the past on computer vision, distributed and embedded systems. Right now, I am a PhD Student at the University of Colorado Boulder working on Human Robot Interaction research and working on the development of Autonomous Vehicles at NVIDIA. I completed my undergrad at the University of Illinois at Urbana-Champaign in Computer Engineering and my masters in Computer Science and Robotics at USC.
Academic and Professional Timeline
- 2024-Present / University of Colorado Boulder - HIRO Lab / PhD Student advised by Professor Alessandro Roncone
- 2022-2023 / University of Southern California - ICAROS / Graduate Research Assistant under Professor Stefanos Nikolaidis
- 2021 / New York University - Game Innovation Lab / Graduate Research Assistant under Professor Julian Togelius
- 2018-Present / NVIDIA / Automotive Deep Learning Solution Architect
- 2017 / NVIDIA / Automotive Deep Learning Solution Architect Intern
- 2016 / University of Illinois Urbana-Champaign - Bretl Research Group / Undergraduate Research Assistant under Professor Tim Bretl
- 2016 / Nest Labs / Embedded Software Engineering Intern
- 2014 - 2018 / University of Illinois Urbana-Champaign - Vision Group / Undergraduate Research Assistant under Professor Derek Hoiem
- 2013 - 2014 / University of Colorado Boulder - Correll Lab / High School Research Assistant under Professor Nikolaus Correll
Most Recent Papers, Posters and Preprints
-
[IEEE RA-L] Selecting Source Tasks for Transfer Learning of Human Preferences
We address the challenge of transferring human preferences for action selection from simpler source tasks to complex target tasks. Our goal is to enable robots to support humans proactively by predicting their actions — without requiring demonstrations of their preferred action sequences in the target task. Previous research has relied on human experts to design or select a simple source task that can be used to effectively learn and transfer human preferences to a known target. However, identifying such source tasks for new target tasks can demand substantial human effort. Thus, we focus on automating the selection of source tasks, introducing two new metrics. Our first metric selects source tasks in which human preferences can be accurately learned from demonstrations, while our second metric selects source tasks in which the learned preferences, although not as accurate, can match the preferred human actions in the target task. We evaluate our metrics in simulated tasks and two human-led assembly studies. Our results indicate that selecting high-scoring source tasks on either metric improves the accuracy of predicting human actions in the target task. Notably, tasks chosen by our second metric can be simpler than the first, sacrificing learning accuracy but preserving prediction accuracy.
-
[PyTorch Conference 2022] Torch-TensorRT: A Compiler for Accelerating PyTorch Inference Using TensorRT
Torch-TensorRT is an open-source compiler targeting NVIDIA GPUs for high-performance deep-learning inference in PyTorch. It combines the usability of PyTorch with the performance of TensorRT allowing for easy optimization of inference workloads on NVIDIA GPUs. Torch-TensorRT supports all classes of optimizations in TensorRT including reduced mixed precision down to INT8, through simple Python & C++ APIs designed to work directly from PyTorch. Torch-TensorRT outputs standard PyTorch modules as well as the TorchScript format to allow for a completely self-contained, portable, & static module with TensorRT engines embedded as attributes
-
[PyTorch Developer Conference 2021] Torch-TensorRT: Accelerating Inference Performance Directly from PyTorch using TensorRT
Torch-TensorRT is an open-source SDK designed to target NVIDIA GPUs for high-performance deep-learning inference. It combines the usability of PyTorch with the performance optimizations of TensorRT allowing for easy optimization of any inference workload on NVIDIA GPUs. Torch-TensorRT supports all optimizations of TensorRT including reduced mixed precision down to INT8, all within simple Python & C++ APIs designed to work directly from PyTorch. Torch-TensorRT outputs the standard TorchScript format to allow for a completely self-contained, portable, & static module with TensorRT engines embedded as attributes. Torch-TensorRT partitions the model into subgraphs based on TensorRT compatibility of each node. Compatible subgraphs are replaced with a single optimized TensorRT engine; the incompatible subgraphs “fallback” and run in native PyTorch. This fallback means there is no requirement for full model support by TensorRT, expanding Torch-TensorRT compatible models to all of TorchScript.
Most Recent Talks
-
[GPU Technology Conference Fall 2021] Accelerate PyTorch with TensorRT
Learn how to accelerate PyTorch inference without leaving the framework with Torch-TensorRT. Torch-TensorRT makes the performance of NVIDIA’s TensorRT GPU optimizations available in PyTorch for any model. You'll learn about the key capabilities of Torch-TensorRT, how to use them, and the performance benefits you can expect. We'll walk you through how to easily transition from a trained model to an inference deployment fine-tuned for your specific hardware, all with just a few lines of familiar code. If you want more technical details, the second half of the talk will give you a chance to deep dive into how Torch-TensorRT operates, the mechanics of key features, and a few in-depth examples.
-
[GPU Technology Conference Spring 2021] New Features in TRTorch, a PyTorch/TorchScript Compiler Targeting NVIDIA GPUs Using TensorRT
We'll cover new features of TRTorch, a compiler for PyTorch and TorchScript that optimizes deep learning models for inference on NVIDIA GPUs. Programs are internally optimized using TensorRT but maintain full compatibility with standard PyTorch or TorchScript code. This allows users to continue to feel like they're writing PyTorch code in their inference applications while fully leveraging TensorRT. We'll discuss new capabilities enabled in recent releases of TRTorch, including direct integration into PyTorch and post-training quantization.
-
[GPU Technology Conference Fall - Oct, 2020] TRTorch: A PyTorch/TorchScript Compiler Targeting NVIDIA GPUs Using TensorRT
We'll dive into TRTorch, a new compiler for PyTorch and TorchScript that optimizes deep learning models for inference on NVIDIA GPUs. Programs are internally optimized using TensorRT but maintain full compatibility with standard PyTorch or TorchScript code. This allows users to continue to feel like they're writing PyTorch code in their inference applications while fully leveraging TensorRT. We'll cover how the compiler works internally and different ways to leverage the compiler.
Most Recent Posts
-
Understanding the Waypoint Transformer
Supervised goal conditioned reinforcement learning using generated intermediate goals.
-
Building CLIP from Scratch to Classify Pokemon
Learning about how Contrastive Language-Image Pre-training (CLIP) works
-
All Recommendation Engines Should Follow Twitter's Example on Transparency
Twitter released the source for its recommendation engine to be transparent about what it promotes and suppresses. Others should be required to do the same.